
Projects
Operationalizing Drone Imaging Technology to Detect Nutrient Deficiencies in Fruit Orchards

Active
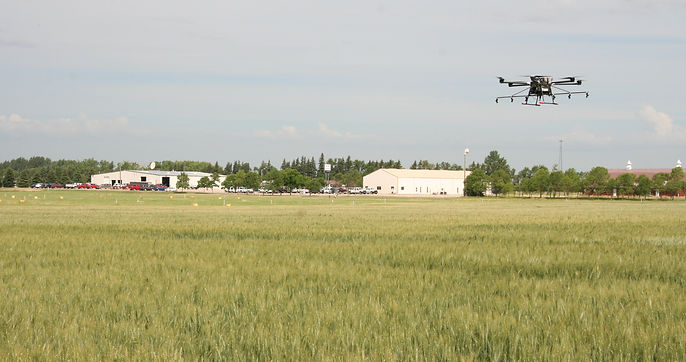
Project Summary
Our project seeks to operationalize a novel technology that has been developed over the last three years, which has tied drone imaging to the detection of plant nutrient statuses on a whole-farm scale. The technology relies on a number of predictive models, based on correlations found between two drone imagery derived vegetative indices (NDVI, NDRE) and individual internal plant macro- and micronutrient levels, to accurately determine the need for supplemental nutrient applications within fruit orchards. This project has three goals, together which would allow for its adoption by our local agricultural community: 1) determine if corrective action based solely on the drone models affects fruit yield and/or fruit quality, 2) determine if the inclusion of super- or suboptimal data points from less experienced growers extend the effective range of the models, and 3) if the technology is economically feasible, affording cost savings onto farmers. Over three years, 7 farms will participate in the research process as actively contributing members of the research team. Growers will be engaged with on-farm demonstrations and will be responsible for applying recommended corrective actions as well as participating in the evaluation of the treatments on fruit yield and quality. We have designed this study to include and engage historically underserved farmers in our community in an effort to pursue social justice.
Objectives
Validate predictive models utilizing drone images and derived vegetation indices (NDVI, NDRE) to accurately predict plant tissue nutrient levels on a whole-farm scale, aiming to replace traditional and costly tissue analysis methods.
Operationalize the validated predictive models to enable quick and reliable prediction of plant tissue nutrient levels for apple, peach, blueberry, and grape crops, encompassing both macro- and micronutrients.
Implement farmer-centric corrective actions, such as foliar sprays, solely based on model-based predictions, with control plots established for comparison to ensure efficacy.
Utilize three years of comprehensive data across three locations in Connecticut to develop and refine the predictive models for optimal accuracy and reliability.
Verify the effectiveness and practicality of the developed technology with growers, aiming to facilitate its adoption and replacement of existing nutrient-testing practices within agricultural operations.
Funding Agency
Northeast Sustainable Agriculture Research and Education
Project Members

Chandi Witharana (PI)
University of Connecticut
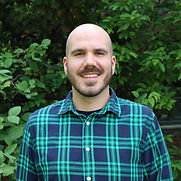
Evan Lentz
University of Connecticut
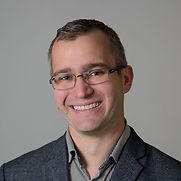
Sandro Steinbach
North Dakota State University